Automatic model-based teeth segmentation, numbering and 3-D reconstruction using routinely collected images
Oral health issues like tooth decay affect billions of people worldwide. The early detection as well as the treatment of those problems is an important part of today's healthcare. Since the teeth cannot be assessed by visual inspection only as they are partly embedded in soft tissue and bones, medical imaging techniques are employed to provide the required information. The manual analysis of such images is time consuming and prone to inter- and intra-observer variability. For this reason, computer aided systems which automate the extraction of clinically relevant information can be of great benefit to medical professionals. A mandatory step to provide such systems is the segmentation and numbering of individual teeth in the digital images. However, challenges like image quality and characteristics of dental radiographs, patient-specific variations, and the fact that the 32 teeth only belong to 4 different types makes it hard to accurately detect teeth boundaries and difficult to label an individual tooth.
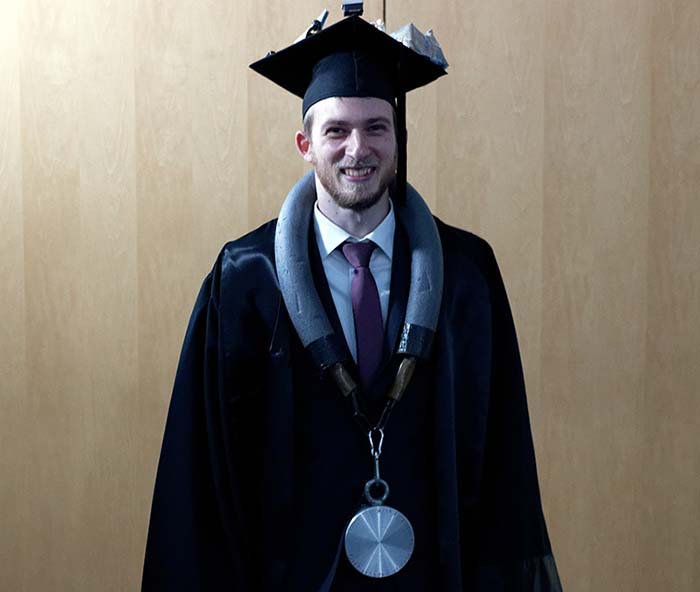
In his dissertation, which he successfully defended on December 20, 2021, Andreas Wirtz, research associate in the department "Visual Healthcare Technologies", describes how computer-aided methods could replace the time-consuming manual analysis - congratulations!
The public defense of the dissertation on "Automatic model-based teeth segmentation, numbering and 3-D reconstruction using routinely collected images" took place on December 20 at Fraunhofer IGD in Darmstadt and online. Supervisors of the thesis were Prof. Dr. techn. Dieter W. Fellner (TU Darmstadt), Prof. Dr. Arjan Kuijper (TU Darmstadt) and Prof. Dr. Reinhard Klein (University of Bonn).
Abstract
In this context, this thesis focuses on the research questions of teeth segmentation and numbering in panoramic X-ray images, and image-based 3-D reconstruction of the teeth from five colored photographs which relies on accurate object-level segmentation and numbering of the 2-D teeth outlines (in the photographs). As both topics share the common aspect of segmentation and numbering, a general concept is presented which is also applied in a third application to localize landmarks for the analysis of dental cephalometric images.
This thesis proposes to solve the segmentation and numbering in 2-D by encoding prior knowledge about the teeth shapes and spatial relations in a coupled shape model. Initial placement of the model is performed by exploiting the semantic segmentation performance of neural networks while dynamic adaptation strategies increase the robustness of fitting to model to unseen images. This enables the extraction of teeth contours from both panoramic radiographs and colored photographs. The proposed image-based 3-D teeth reconstruction utilizes the numbered teeth contours from the photographs to deform a mean model of the teeth by minimizing a silhouette-based loss. It is the first fully-automatic image-based teeth reconstruction that aims to reconstruct the majority of teeth and the first approach to perform a reconstruction only on the five photographs of orthodontic records. The landmark localization utilizes the segmentation and numbering concept to predict the location of 19 landmarks by exploiting the spatial relation between landmarks and other structures and refines those predictions using landmark-specific Hough Forests.
The teeth segmentation and numbering of 28 individual teeth in panoramic radiographs achieves average F1 scores of 0.823 ± 0.189 and 0.833 ± 0.108 on two different test sets. The image-based 3-D reconstruction of 24 teeth from five photographs achieves an average symmetric surface distance of 0.807 ± 0.379 mm. The landmark localization in cephalometric images reaches a success detection rate of 76.04 % in the clinically relevant 2.0 mm accuracy range.