Using municipal land more sustainably
Across Germany, local government agencies are hoping to use far less virgin land for local housing developments by the end of the decade. To identify suitable plots within existing towns and cities more efficiently and quickly, Fraunhofer IGD is developing a self-learning algorithm.
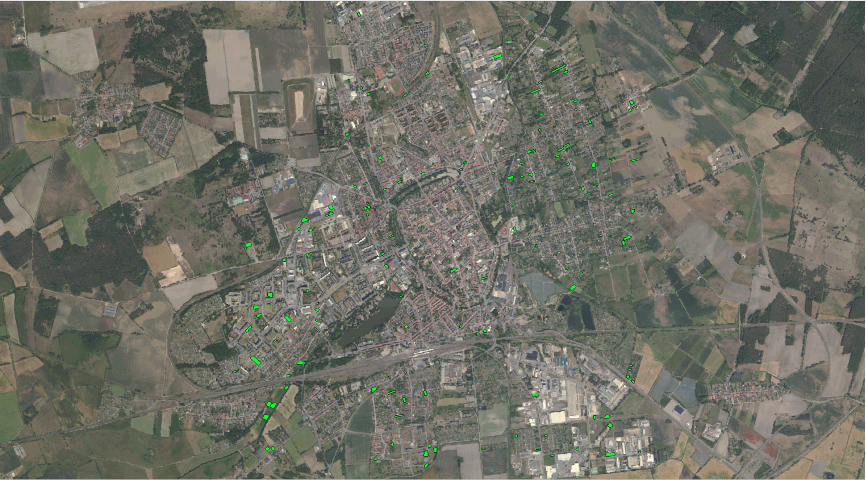
Although land as a resource cannot, strictly speaking, be “consumed,” it is nevertheless finite. Once land has been developed, it is generally unavailable for any other use for many years. This creates major challenges for local urban planners, especially regarding sustainable, future-proof housing. The overall goal is to develop land in a way that takes into account economic, demographic and ecological needs—while using as little space, especially virgin land, as possible.
On-site inspections are costly and time-consuming
It is essential that planners are able to identify plots of unused land within existing developments, areas where population density could be increased, possible in-fill sites, and disused buildings. This prompted Fraunhofer IGD to launch a project in collaboration with the Ministry for Infrastructure and Digital Transformation (MID) of Saxony-Anhalt. Since 2005, the MID has been collecting data on plots of land with development potential, drawing on information in geodata systems and local land registers. This is combined with aerial photographs and data from remote-sensing satellites. These inputs must be carefully checked against defined criteria, and any promising land subsequently verified by a physical inspection.
Self-learning algorithm streamlines the search for land
The Fraunhofer IGD development team aims to make this process far more efficient by combining geoinformation, computer vision and an artificial neural network. A prototype self-learning algorithm is already in use and proving effective. It can autonomously identify plots of land with development potential by means of semantic segmentation. The algorithm assigns a class to every pixel of a given satellite image and compares them with zoning maps. The geodata are sourced from the Web Feature Service (WFS) and the Web Map Service (WMS). The self-learning algorithm continuously gains new insights and fine-tunes its parameters and results—currently delivering an accuracy of 86 percent. This greatly reduces the need for on-site visits, saving time and money.
Once identified, suitable plots of land can then be filtered according to further parameters relevant to urban development goals: for example, which plots are close to streets, major freeways, or train stations? “We have succeeded in developing a novel method of automatically identifying underutilized land on aerial photographs. We hope this will help the State of Saxony-Anhalt to establish a comprehensive register of land with development potential,” emphasizes Tom Krause, project lead at Fraunhofer IGD. Other states and government agencies also stand to benefit from this new technology, with solutions tailored to their local needs and regulations.